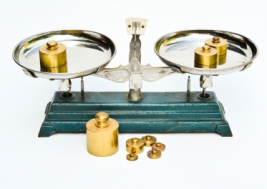
In almost all quantitative studies, the final sample is weighted to better account for the population it seeks to represent. A target population always has specificities, be it in terms of region, age, gender, or any other target characteristic (i.e.: consumption of some sort of yogurt or membership to some financial institution). Although data is collected with the aim of respecting these specific distributions within the sample, it becomes sometimes difficult and / or costly to reach the exact number of respondents within each segment. As such, for reasons of feasibility, compromises are made during the process of data collection, which are later corrected through weighting. So, for example:
- I want to know if blondes and brunettes have different perceptions of a brand of yogurt and to know this I want to speak to 1,000 respondents.
- I estimate their distribution in the general population to be at 80% brunettes and 20% blondes so my perfect sample would be composed of 800 brunettes and 200 blonds.
- But it appears I am better able to reach blonde respondents, so the sample I obtain is made up of 65% brunettes and 35% blondes, or 650 brunettes and 350 blonds.
- I will therefore attribute a weighting factor of 1.23 to all my brunettes and a factor of 0.71 to all my blondes.
So in case blondes had a certain characteristic that could bias the final results, I am not overestimating them in my sample, and therefore not accounting for that difference more so than what it naturally is in the population.